To make best use of your data asset across your organization, it is essential to have a solid data quality framework to ensure that your data is accurate and complete. But all data quality management models are not created equal: different organizations have different levels of data quality maturity, depending on organizational priorities and needs.
Data quality maturity model
To get your organization to a level of data quality that helps support data that is fit for purpose, evaluate your framework against the data quality maturity model. This model provides a benchmark for assessing your company’s processes around data management and data quality, to determine areas of risk and identify areas where additional investment may be needed.
There are four key stages of data quality sophistication:
- Unaware: These organizations are unaware of the importance of data quality and its impact on the business. There is a sense of apathy towards the issue of data quality, and no initiatives are in place to help tackle problems. Data is seen as "good enough" and workarounds are implemented when needed.
- Reactive: These organizations react to data quality issues as they impact business performance, but there is no sophistication when dealing with data at a corporate level. Tools and processes are typically very tactical, or point solutions that live within department silos. Investments in data quality are typically in response to specific, compelling events in the short term, like a compliance breach.
- Proactive: These organizations have clear, proactive data management strategies, with some defined roles and responsibilities around data within the business. Data processes are better understood across departments, and silos have begun to be broken down, allowing for collaboration between IT and business users. The value of data assets is being realized. There is a focus on profiling data issues and seeking out and solving or root causes of problems.
- Optimized: These organizations have developed a fully governed data quality environment, where data quality is monitored with documented rules in place. Data has a single owner that is responsible for corporate-wide data management, consolidating their processes and technology investment. They can identify and communicate the link between data quality and financial performance.
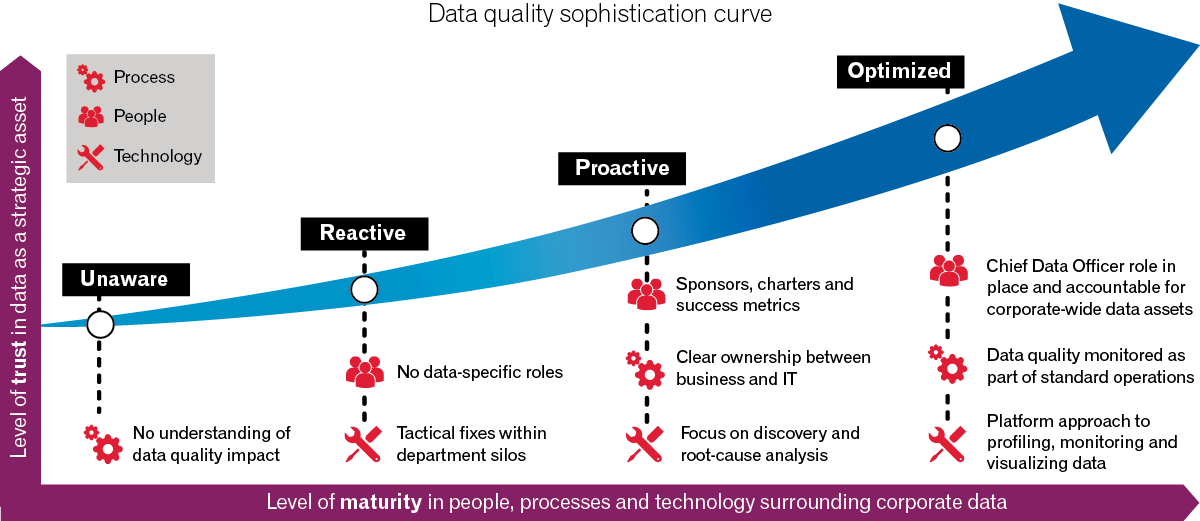
How mature is your data quality framework?
While 88 percent of companies have some sort of data quality strategy in place, many are in early stages of sophistication. Only 18 percent of companies say they have reached the optimized state of data quality. By contrast, 43 percent of companies perform data analysis and cleansing only as issues arise.
Assessing where your organization fits on this model can help you determine what the next best steps are in building your data management practices, so your company can trust that its data asset can drive good business decisions.
To determine where your business sits on the data quality maturity model, you need to consider the following areas:
People
People are the critical component to any effective and robust data quality strategy. Having the right people in place to champion and implement your data management practices are essential to creating a business culture that supports data quality as status quo. To assess what your people investment in data quality should be, ask questions like:
- Who owns data within your organization? Is it centrally managed or is it held across various business silos?
- Does your business have a clear data quality strategy in place or are issues handled on an ad hoc basis?
Process
Your processes are designed to clearly identify data quality roles and responsibilities. Without process in place, quality issues will inevitability unfold as the volume of data you handle increases. Consider taking a look at:
- How are data issues uncovered? Through monitoring and resolution? Or as they happen with workarounds?
- Does your organization have measures in place to report on data quality proactively?
Technology
Data quality technology has evolved over recent years and involves more than just point solutions; they now offer critical support for managing information across its full lifecycle, from data discovery through to monitoring and visualization. Evaluate your company’s use of data management technology by asking:
- How reliant is your organization on manual tools and techniques when solving data quality issues?
- When investing in data quality technology, how broadly is it used across the organization?
By determining where your organization lies on the data quality maturity model, you can determine what your next best steps and areas for investment are in creating a robust data quality framework, and ultimately driving better quality data for better decision making.
Learn more about how you can create a more sophisticated strategy by reading our paper on creating an ideal data quality framework.
Download now